Harnessing AI Search: Transforming Information Retrieval for the Modern User
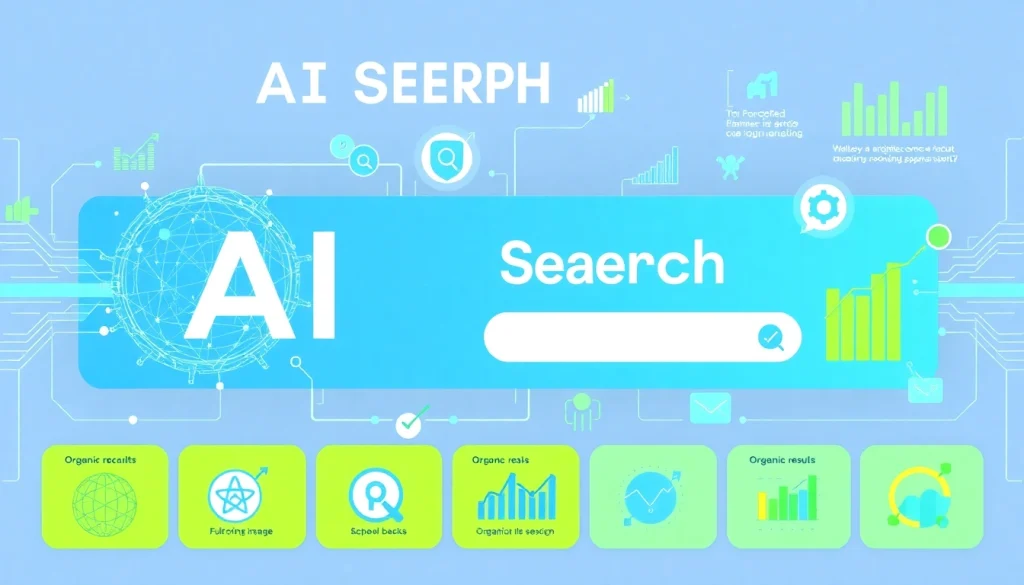
Understanding AI Search Technology
What is AI Search?
AI search refers to the utilization of artificial intelligence technologies to enhance the process of searching for information. Unlike traditional search engines that principally rely on keyword matching and indexing, AI search engines employ machine learning, natural language processing (NLP), and algorithms to interpret user queries and deliver results that are more contextually relevant. AI search understands the intent behind queries and can respond to complex questions that may involve multi-faceted concepts.
This evolution of search technology aims to provide users with a more interactive and intuitive experience. For example, AI search systems can generate synthesized answers from vast data pools, offering more than just links to various sources. They create a bridge between human language and machine understanding.
Key Features of AI Search Engines
- Natural Language Processing: This enables AI search engines to comprehend and process human language, which helps in deciphering the intent behind a query and providing answers in a more conversational manner.
- Contextual Understanding: AI search systems can analyze the context of queries, taking into account previous interactions and user profiles to deliver tailored results.
- Real-time Updates: AI search algorithms continuously learn from user interactions and data inputs, allowing them to provide the most current information available.
- Image and Voice Search: Advanced AI search engines encompass features that allow users to search using images or voice commands, making the search process more versatile.
- Answer Synthesis: Rather than merely listing links, AI search engines compile and synthesize information from multiple sources to present concise answers directly within the search results.
Comparison with Traditional Search Engines
Traditional search engines operate primarily through algorithms that index web pages based on keyword relevance. While effective for straightforward searches, they often struggle with contextual nuances. In contrast, AI search engines are designed to offer a more holistic search experience.
For instance, when a user searches for “best practices for remote work,” a traditional search may return a list of articles featuring this phrase. An AI search engine, however, will consider user intent and may provide summaries, tools, or strategies tailored for remote work, often citing the most relevant sources rather than merely presenting them.
Benefits of Implementing AI Search
Improved Relevance in Results
One of the primary advantages of AI search is its ability to deliver results that align closely with user intent. By leveraging deep learning techniques, AI search understands the context and nuances of queries, enabling it to discern variations in language and phrasing.
For instance, if a user queries “how to improve team productivity,” AI search engines can provide results that specifically address techniques, tools, and strategies tailored to various team structures and industries. This enhances the likelihood of user satisfaction as their specific needs are met with precision.
Enhanced User Engagement
User engagement significantly improves when provided with relevant and timely information. By employing AI search, organizations can create an environment that encourages exploration and interaction. Features such as predictive text and suggested searches guide users toward related content, keeping them engaged longer.
Moreover, personalized recommendations based on previous search behavior foster a sense of tailored service. For example, a user seeking articles on digital marketing may receive suggestions for related topics or new publications that align with their interests.
Time-Saving Search Solutions
AI search engines are designed to return results quickly, effectively minimizing the time users spend looking for answers. By synthesizing information and presenting concise summaries in response to complex queries, they streamline the search journey.
For professionals and researchers, this capability can save hours of searching. Instead of sifting through multiple links, users receive synthesized information that answers their inquiries directly, thus enabling them to focus on implementing their findings rather than searching for them.
Challenges in AI Search Implementation
Data Privacy Concerns
While AI search engines are designed to enhance the user experience, they often utilize personal data to refine their algorithms and personalize results. This data dependency raises significant privacy concerns among users. Organizations must navigate the balance between personalization and privacy carefully.
To address these challenges, companies can implement robust data protection policies, ensuring users are informed and have control over their data. Employing transparency in data usage can build trust with users, fostering a better relationship with AI technologies.
Accuracy and Reliability Issues
AI search engines are only as good as the data they process. Inaccurate or incomplete data can lead to misleading search results. Furthermore, biases present in the training datasets can influence AI behavior, resulting in skewed outputs.
To counteract these challenges, ongoing audits and data quality assessments should be conducted. Continuous training with diverse datasets will enhance the accuracy and reliability of AI outcomes. External validation checks and algorithm transparency are also crucial in ensuring responsible AI deployment.
User Trust and Acceptance
As with any new technology, user acceptance plays a vital role in the success of AI search engines. Some users may feel skeptical about the accuracy and appropriateness of AI-generated content, fearing it replaces human judgment.
Launching awareness campaigns that educate users on the benefits and workings of AI search technology can significantly enhance acceptance. Providing options for users to customize their search experiences can also create a sense of ownership and comfort.
Future Trends in AI Search
Advancements in Natural Language Processing
The continuous evolution of NLP technology is setting the stage for more sophisticated AI search systems. Future advancements will enable AI to understand and respond to language subtleties, regional dialects, and even emotional tones. This will lead to exponential improvements in AI-driven interactions.
AI search engines are expected to integrate multi-language support seamlessly, allowing users from diverse linguistic backgrounds to leverage AI technologies effectively. By closely mimicking human conversational patterns, AI will cater to a broader audience.
Integration with Other AI Technologies
As AI technologies mature, the integration of AI search engines with other AI-driven tools will become common. For instance, combining AI search with recommendation systems can create a holistic learning environment. As users search, they may receive suggestions for related resources or tools tailored to their inquiries.
This interconnectedness will facilitate greater collaboration among AI systems, enhancing the overall user experience and promoting deeper learning and engagement.
The Role of Personalization
The significance of personalized search experiences will increase as AI search technologies evolve. Future AI search engines will utilize a user’s historical search behavior and preferences to curate unique search experiences, almost akin to having a personal search assistant.
This personalization extends beyond just showing relevant content; it will also consider time-zone preferences, location-based queries, and even the user’s recent actions. Such a tailored approach promises to resonate more with users, making AI searches feel more human-like and relatable.
Best Practices for Using AI Search Effectively
Optimizing Content for AI Search Engines
For organizations looking to leverage AI search, optimizing content is vital. This includes ensuring clarity of language, using appropriate keywords, and structuring content hierarchically to facilitate better AI interpretation.
Moreover, employing schema markup can help define the nature of content clearly, aiding AI search algorithms in understanding context. Regularly updating content to keep it relevant also plays a crucial role in maintaining high search visibility.
Leveraging User Feedback
User feedback is invaluable in refining AI search experiences. Engaging users through periodic surveys regarding their search experiences can uncover areas for improvement and highlight aspects that are functioning successfully.
Similarly, analyzing user interactions and adjusting algorithms based on this feedback can enhance the effectiveness of search results. Creating feedback loops enables continuous learning and enhancement for AI systems.
Continuous Improvement Strategies
The landscape of AI technology and user needs is constantly evolving. Organizations must adopt continuous improvement strategies, regularly reassessing and optimizing AI algorithms based on new trends, data inputs, and user behaviors.
Incorporating iterative testing, exploring new AI methodologies, and strong partnerships with AI research institutions can foster innovation. Staying committed to enhancing AI search technology reflects the values of adaptability and forward-thinking innovation within an organization.